The most recent trends report from Action Fraud makes grim reading. In 2020/21, over 875,000 identity theft incidents were reported within the UK, compared to around 750,000 reports two years earlier. Losses topped £2.3bn.
The Office for National Statistics (ONS) says that people are more likely to fall victim to fraud or cyber offences than any other crime.
The sheer level of fraud means that banks, insurance companies, e-commerce, and alike face huge reputational risks if they fail to take identity theft seriously.
Table of Contents
ToggleThe biometric sweet spot
Unfortunately, protecting against identity theft is becoming increasingly more difficult.
Security-conscious businesses have implemented more challenging password protocols and two-factor authentication over recent years to compensate; however, data breaches are still common. With the right technology, an eight-letter password can be cracked in 12 minutes or less.
Today’s fraudsters have never had it so easy, with a plethora of personal information on social media and also available for sale on the dark web, such as account numbers, date of birth, PINs, passwords, addresses, etc.
Many businesses understand these threats but struggle to find the right balance between security and customer experience. Customers can quickly become frustrated with cumbersome log-in processes and onboarding journeys that are too complex, putting them off altogether. There’s plenty of evidence that a massive chunk of potential app users, for example, fail to make it past onboarding, and the churn rate can be as much as 60 – 70%.
Biometric identification has the potential to hit the sweet spot between security and usability. It uses face, voice, fingerprint or palm recognition to prove someone’s identity in seconds. And because biometrics focuses on our unique human characteristics, it’s more difficult to defraud.
Facial recognition and the rise of the deepfake
Facial and voice identity verification are increasingly popular because they can accurately prove someone’s identity in a mere second while reducing fraud.
As biometric technology gains popularity, so do the fraudsters’ attempts to break it; thus, the rise in deep fakes.
A deep fake is a synthetic image, video or voice produced by machine learning processes that appear authentic, aiming to take someone’s identity to commit a crime. A deep fake can be produced in real-time, from the original to the reconstructed.
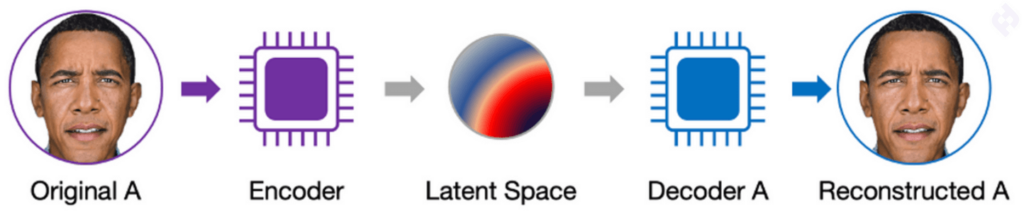
Deep fakes have been used to put words in Obama’s mouth, therefore could easily be used to rob your customers and defraud your organisation.
Looking for the truth – deepfake detection
The ability to manipulate images and video has been around for years, but while the results were undoubtedly fake, they indeed weren’t deep. It was easy to spot a fraud.
Not anymore. Relatively inexpensive software can now create realistic-looking deep fakes. All a fraudster needs are a collection of photos or video clips of the person they want to imitate, which they can often find on Facebook and Instagram. The AI will do the rest.
The dilemma for businesses is that customers like the ease of using facial recognition, but many solutions are easily fooled by deep fakes.
That’s a massive concern because banks and other financial services businesses rely on biometric authentication. Fraudsters can potentially open accounts, apply for loans and credit cards and transfer money using deepfake technology.
With that in mind, the need for sophisticated deepfake detection is becoming critical.
Deepfake prevention through liveness detection
So, what can businesses do to distinguish real customers from a fraudster’s manipulated image or video?
The key is an extra layer of facial and voice recognition technology called liveness detection. This liveness check can detect if the person in front of the camera is real (they are a live person, and their image matches their validated ID).
Meanwhile, voice liveness detection can distinguish the live, genuine voice from recorded or mimicked voices and synthesised voice deep fakes.
These ‘presentation attack detection (PAD) systems use sophisticated AI to distinguish video and voice replays from live interaction.
They create a kind of two-factor authentication for biometrics. Facial recognition software detects if the facial details of a live image match those on a stored picture. Liveness detection then decides if the live image is of a physically present person at that moment.
What is active liveness detection?
Active liveness detection technology requires the end-user users to look into the camera and perform gestures or movements such as turning the face from left to right, looking up and down, blinking, or zooming the camera in and out. As a result, this approach requires users to spend more time and effort when onboarding, resulting in higher abandonment rates.
Furthermore, active liveness is not best suited for authentication as the process of looking up and down etc., causes significant friction.
Active liveness usually does not utilize any sophisticated machine learning model, and they might be vulnerable to attacks such as silicon masks.
What is passive liveness detection
Passive liveness requires no active user participation, no movements, gestures, smiling, blinking, head-turning, zooming, or moving the camera; it simply runs in the background, thus frictionless.
Passive liveness technologies rely on Machine Learning, and they are not vulnerable to presentation attacks using silicon masks.
Active Liveness vs Passive Liveness Detection
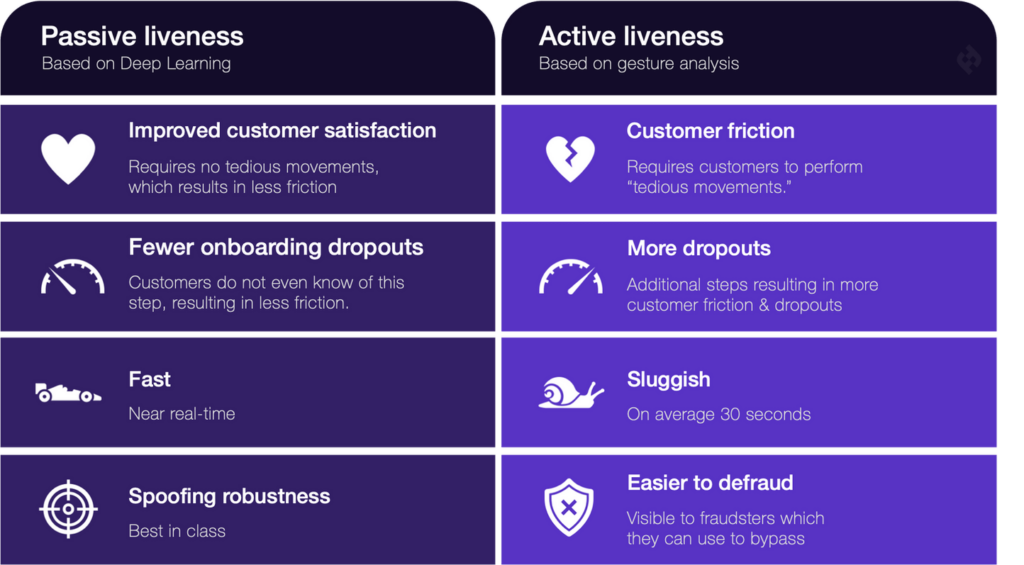
What to look for in presentation attack detection
In other words, face recognition technology alone isn’t enough. Adding a liveness detection layer to your authentication process is the best detection against deep fakes.
But which one? Well, ISO-30107 is the gold standard for presentation attack detection and face recognition systems. Solutions that meet this standard are tested by iBeta, an accredited independent software testing lab. There are two levels of facial liveness detection accreditation.
- Level 1 ISO-30107 means the software can detect 2D paper photos (flat and bent), digital photos, and paper masks (flat and bent) worn by an actual human.
- Level 2 ISO-30107 means the software can detect lifelike 3D mannequin heads, realistic masks worn by a person and deepfake videos.
Where do we fit in?
Udentify ID Verification and Face Authentication from Fraud.com enables you to filter out the fakes, tested by iBeta level 1 and level 2 for Presentation Attack Detection (PAD).
Udentify verifies if it’s the right person (not an imposter or criminal) and a real person (not a photograph, video, mask, or digital screen).
Our facial liveness detection layer is passive, so users won’t even know it’s happening. With 99.9% accuracy, our identity proofing and authentication is a frictionless way to guard against the threat of deepfake technology.
Bye-bye fraudsters. And hello, real live customers.
Learn more about Udentify